The Ultimate Guide to Machine Learning Labeling Tools
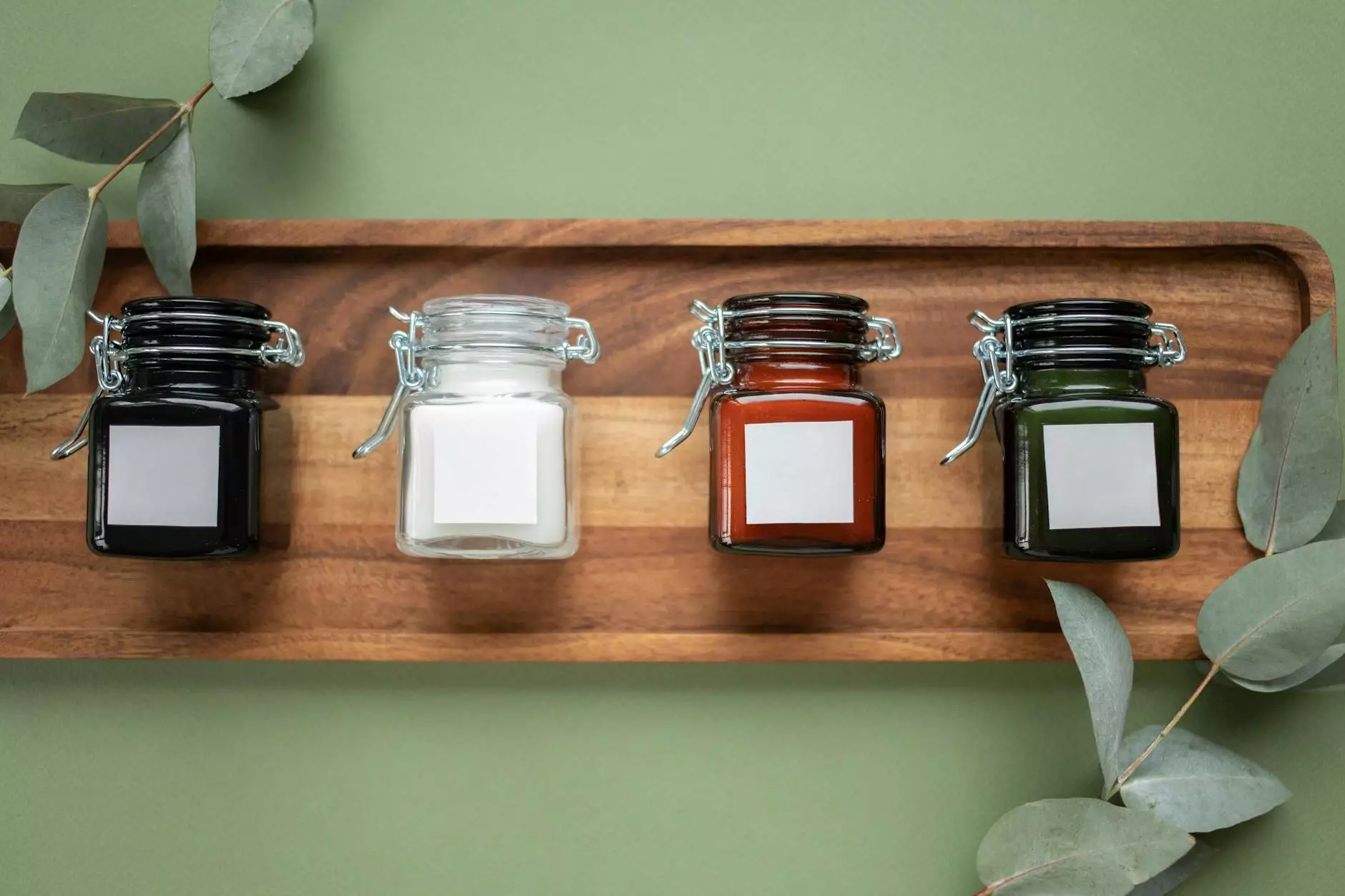
Machine learning labeling tools are at the forefront of transforming how businesses leverage data to enhance decision-making, streamline operations, and innovate their product offerings. In today's data-driven world, the role of accurate data annotation cannot be overstated. This article delves into the significance of these tools, especially as they pertain to platforms that offer data annotation solutions.
Understanding Machine Learning Labeling Tools
At its core, a machine learning labeling tool is designed to assist in the annotation of datasets. Annotation is the essential process of tagging or labeling data, which is crucial for training machine learning models. The quality of the annotations directly impacts the performance of these models, making the choice of an effective labeling tool a pivotal decision for businesses.
Why Labeling is Crucial?
In the machine learning pipeline, labeling serves several critical functions:
- Data Quality Improvement: Accurate labels enhance the quality of the training data, resulting in better model accuracy.
- Model Training: Labeled data is essential for training supervised learning algorithms, wherein the algorithm learns to map inputs to outputs based on labeled examples.
- Innovation Enablement: Well-annotated data can uncover patterns and insights that drive business innovation and competitive advantage.
Features of Effective Machine Learning Labeling Tools
When looking for a machine learning labeling tool, several features are crucial for ensuring it meets the diverse needs of businesses:
1. User-Friendly Interface
A tool must offer an intuitive interface, which allows users, regardless of technical expertise, to easily annotate data. A well-designed dashboard enhances productivity by simplifying workflows.
2. Versatility Across Data Types
Data comes in various forms—images, videos, text, and audio. An effective labeling tool should support multiple data formats, allowing businesses to annotate diverse datasets seamlessly.
3. Automation Capabilities
Advanced labeling tools incorporate machine learning algorithms to automate parts of the labeling process. Automation significantly reduces time and costs associated with data preparation, yielding quicker insights.
4. Collaboration Features
In many organizations, labeling tasks are distributed among multiple teams. Therefore, a robust labeling tool should feature collaboration functionalities to facilitate teamwork, enhance communication, and manage tasks efficiently.
Benefits of Utilizing Machine Learning Labeling Tools
The integration of machine learning labeling tools into business operations offers a myriad of benefits, including:
- Enhanced Efficiency: With automated features, the time required for data preparation can be drastically reduced, leading to faster product development cycles.
- Reduction in Errors: Automated solutions minimize human errors, ensuring higher data integrity and reliability.
- Scalability: As businesses expand, so does the volume of data generated. Quality labeling tools are scalable and can handle increased annotation workloads without compromising performance.
- Cost-Effectiveness: By streamlining the annotation process and reducing manual efforts, businesses can achieve significant savings in operational costs.
The Role of Keylabs.ai in Data Annotation
Keylabs.ai stands out as a pioneering data annotation platform that embraces state-of-the-art machine learning labeling tools. With its commitment to providing high-quality labeling services, Keylabs.ai equips businesses with the tools necessary to transform raw data into actionable insights.
Strategies for Effective Data Annotation with Keylabs.ai
To optimize the use of Keylabs.ai’s platform, businesses can follow these strategies:
- Define Clear Objectives: Start by clearly defining the goals of your data annotation project. Understand what you need the model to learn and the type of output desired.
- Choose the Right Tool: With various labeling tools available, choose the one that aligns with your project needs. Whether it’s image, text, or video annotation, select accordingly.
- Train Annotators: Invest time in training annotators on the guidelines, ensuring consistency and accuracy in labeling across the board.
- Regular Quality Checks: Implement a process for regular quality assurance checks to maintain high standards in data labels.
The Future of Machine Learning Labeling Tools
The landscape of machine learning labeling tools is continually evolving. Innovations in artificial intelligence and automation are revolutionizing how data is annotated. Key trends shaping the future include:
1. Integration of AI and NLP
AI is increasingly being utilized to enhance labeling processes, particularly in natural language processing (NLP) tasks. This integration allows for faster and more accurate text annotations.
2. Crowd Annotation
Utilizing crowdsourcing methods for data labeling enables businesses to gather a diverse set of annotations, increasing the robustness of the data.
3. Enhanced Data Privacy
As businesses collect vast amounts of data, ensuring data privacy and compliance with regulations becomes paramount. The future tools will likely enhance security measures against data breaches.
Conclusion
In conclusion, adopting a machine learning labeling tool is not just an operational decision; it's a strategic move that can significantly impact a business's ability to glean insights from data. Platforms like Keylabs.ai exemplify how businesses can harness the power of advanced annotation tools to enhance their machine learning capacities. As the demand for high-quality labeled data continues to soar, investing in the right tools will be critical for businesses aiming to maintain a competitive edge.
By embracing these technologies and strategies, organizations can effectively navigate the complexities of big data, leading to improved productivity, enhanced decision-making, and ultimately, sustainable growth.