Image Annotation for Machine Learning: Unlocking the Power of Data
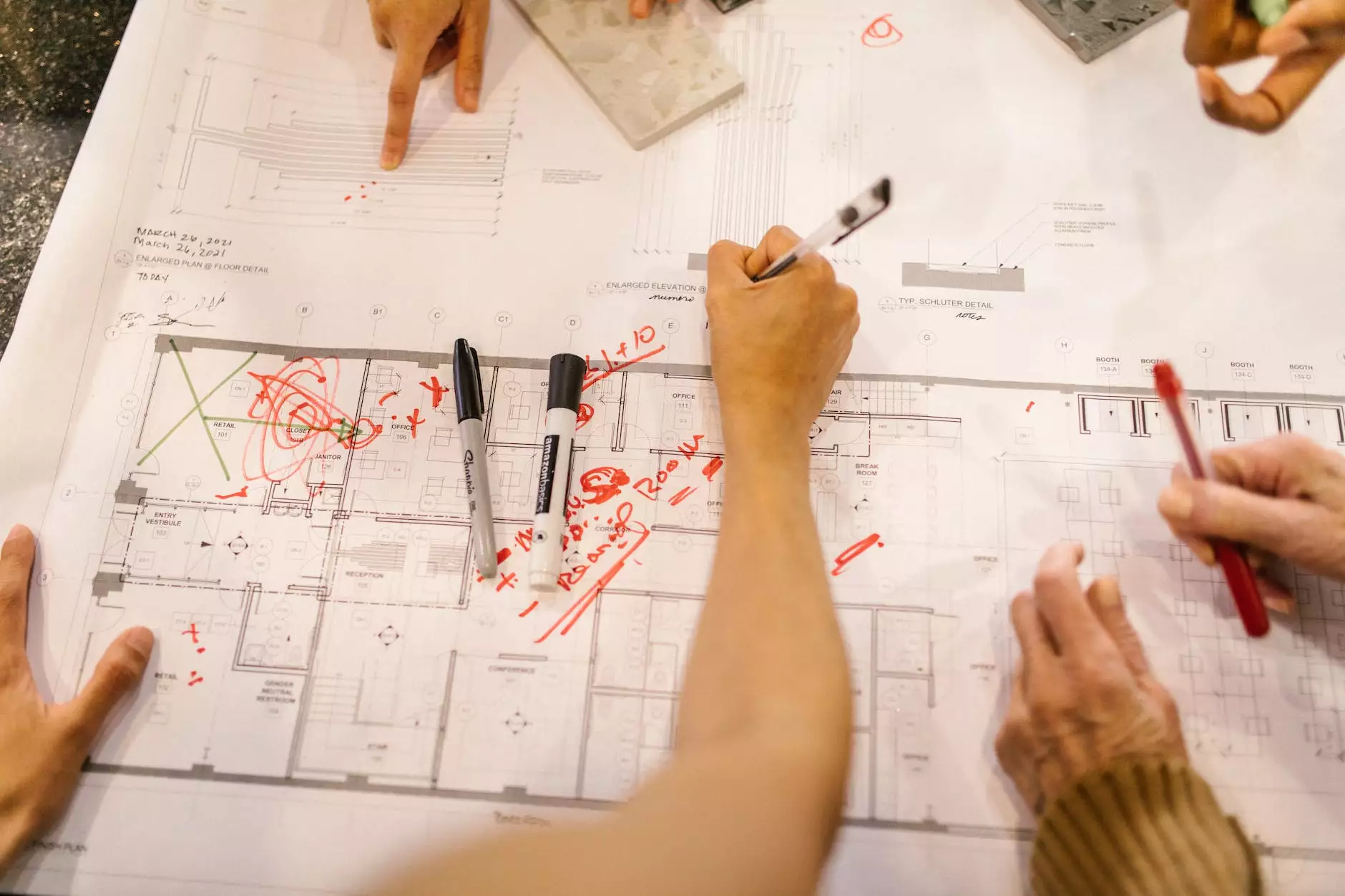
In the era of artificial intelligence and machine learning, the significance of data cannot be overstated. Among the various types of data that fuel these intelligent systems, images hold a crucial place. However, not just any image will do—the images must be properly annotated to ensure effective learning and model accuracy. This is where image annotation for machine learning comes into play.
The Importance of Image Annotation in Machine Learning
As machine learning models are fundamentally reliant on data to learn and make predictions, the quality of that data directly influences the outcome. Image annotation, or the process of labeling images with relevant information, serves as a bridge between raw visual data and machine learning models. Here are some of the pivotal aspects of why this process is essential:
- Enhances Accuracy: Properly annotated images improve the model's ability to recognize patterns, leading to more accurate predictions.
- Facilitates Training: Machine learning algorithms require vast amounts of data to train effectively, and annotations provide the necessary context for understanding.
- Enables Broader Applications: From self-driving cars to medical imaging, annotated images are critical in a wide array of industries.
Key Types of Image Annotation Techniques
Understanding the different types of image annotation techniques is vital for any organization aiming to utilize image data effectively for machine learning. Here are some key techniques:
- Bounding Box Annotation: This involves drawing rectangles around objects in an image. It is one of the most common techniques used in object detection.
- Semantic Segmentation: This technique provides pixel-wise classification, allowing every pixel in an image to be labeled based on the object it belongs to.
- Instance Segmentation: A more advanced version of semantic segmentation, this method differentiates between different instances of the same object class.
- Keypoint Annotation: Used primarily in human pose estimation, keypoints mark specific features on an object or person, such as joints in a human body.
- Polygon Annotation: This method involves outlining complex shapes and is particularly useful for irregularly shaped objects in images.
Challenges in Image Annotation
While image annotation is essential, it is not without challenges. Organizations must be aware of common pitfalls to avoid subpar results:
- High Labor Costs: Annotating large datasets can be time-consuming and costly, especially when relying on human annotators.
- Ensuring Quality: Inconsistent annotations can lead to inaccurate model training, making quality control a critical component of the process.
- Scalability Issues: As the need for annotated data grows, finding a scalable solution becomes imperative.
How KeyMakr Excels in Image Annotation
At KeyMakr, we recognize the paramount importance of quality image annotation for machine learning. Our approach focuses on delivering not only speed but also accuracy, ensuring your machine learning models are built on a solid foundation of well-annotated data. Here’s what sets us apart:
1. Expert Annotators
Our team consists of highly trained professionals who specialize in image annotation. They understand the nuances of various annotation techniques and apply them meticulously.
2. Advanced Tools and Technology
We leverage cutting-edge software that enhances annotation speed while maintaining accuracy. Automation integrated with manual validation ensures the best of both worlds.
3. Scalable Solutions
KeyMakr is equipped to handle projects of any size, whether you need a few hundred images annotated or several thousand. Our scalable solutions accommodate your needs as they grow.
4. Stringent Quality Control
Quality is non-negotiable in machine learning. We implement strict quality control measures, ensuring that every annotation task meets your specifications.
5. Tailored Services
We acknowledge that each project may require a unique approach. Our team is flexible, ready to customize annotation methods that align with your specific objectives.
Applications of Image Annotation in Various Industries
The application of image annotation transcends myriad fields. Below are a few industries where it plays a pivotal role:
1. Autonomous Vehicles
In the automotive industry, image annotation helps train models for object detection and classification, which are crucial for the safe navigation of self-driving cars. By annotating images of pedestrians, road signs, and other vehicles, companies can build robust models that enhance vehicle recognition capabilities.
2. Retail and E-commerce
Businesses in retail utilize image annotation for search optimization and inventory management. Annotated images allow for improved visual search results, making it easier for customers to find products.
3. Healthcare
In medical imaging, image annotation is essential. Accurately labeled images assist healthcare professionals in diagnosing conditions using algorithms trained on annotated datasets.
4. Agriculture
Agricultural technology relies heavily on image annotation for monitoring crop health and detecting potential issues through remote sensing.
Best Practices for Image Annotation Projects
To achieve optimal results in image annotation projects, keep the following best practices in mind:
- Define Clear Goals: Before starting, establish what the end goal of the annotation project is. This clarity will guide the nature of annotations needed.
- Choose the Right Annotation Technique: Selecting the most suitable annotation method is crucial based on your project's specific requirements.
- Invest in Quality Tools: Utilize professional annotation tools that streamline the process while ensuring precision.
- Regularly Review and Validate: Implement checks throughout the annotation process to maintain high standards of quality.
- Seek Feedback: Working closely with your data scientists can ensure the annotations align with the machine learning model's needs.
The Future of Image Annotation in Machine Learning
As technology evolves, the field of image annotation is also set to transform. Emerging techniques such as semi-automated annotation and advancements in machine vision could reduce the dependence on human input, allowing for faster turnaround times and decreased costs. Additionally, as models become increasingly sophisticated, the demand for richer annotations (such as detailed descriptions or context) is likely to rise.
Businesses must adapt to these changes to leverage the full potential of image data. Embracing modern annotation techniques and tools will foster innovation and maintain a competitive edge in the marketplace.
Conclusion: Image Annotation for Success
To conclude, image annotation for machine learning is a fundamental aspect of training algorithms that drive various applications across numerous industries. At KeyMakr, we pride ourselves on providing comprehensive, high-quality image annotation services designed to meet your specific needs. By investing in our services, you gain access to a wealth of expertise, cutting-edge technology, and a commitment to excellence that will help propel your machine learning initiatives forward.
In a world increasingly driven by data, ensuring your annotated images meet the highest standards will ultimately determine the success of your machine learning endeavors. Choose KeyMakr as your partner in navigating this vital aspect of AI, and watch your business thrive!