Unlocking Business Potential with Medical Datasets for Machine Learning
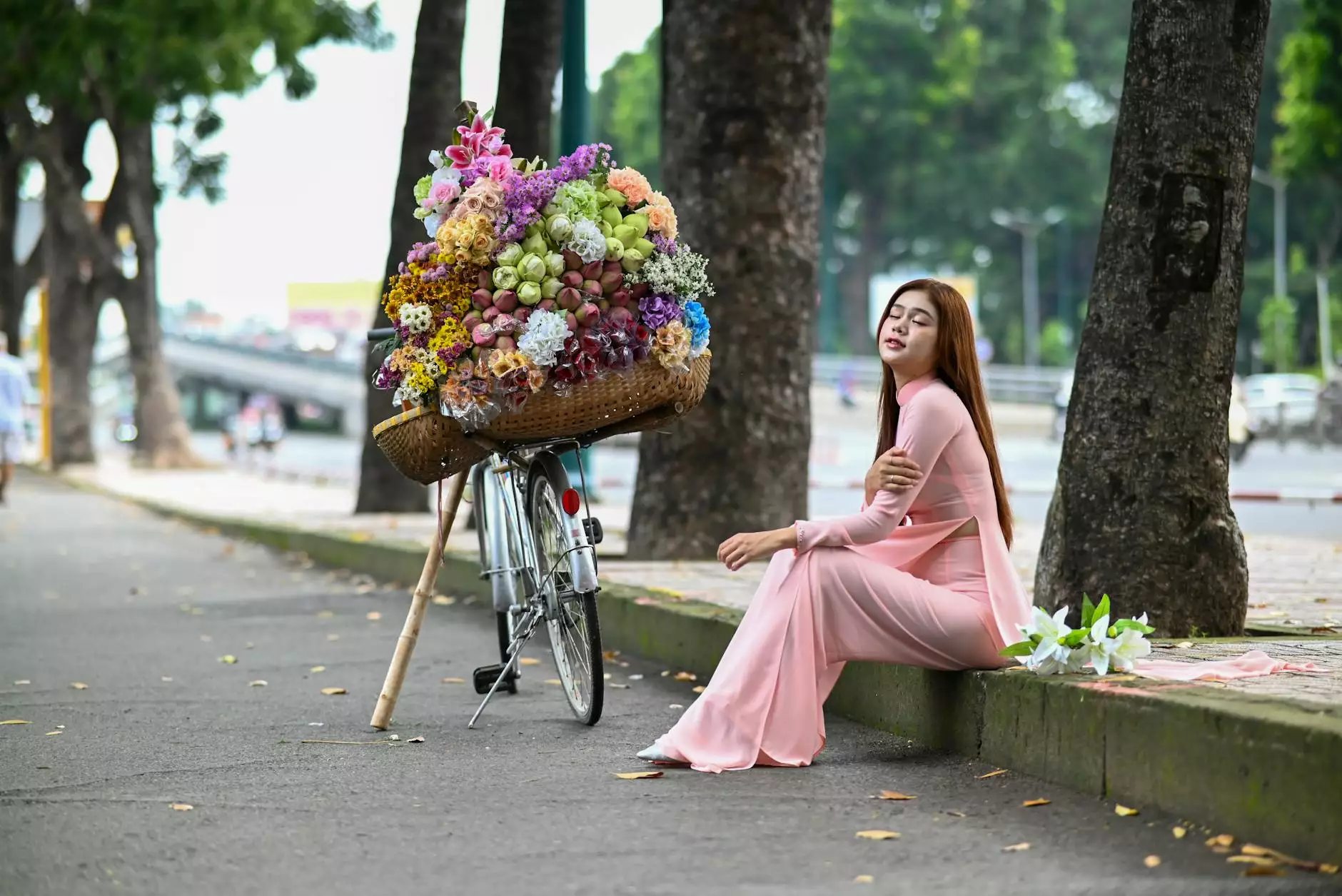
In the ever-evolving landscape of business, innovative technologies such as medical datasets for machine learning are creating opportunities that were once unimaginable. From improving healthcare services to enhancing operational efficiency, businesses are now leveraging data-driven insights to push the boundaries of possibility. In this article, we delve deep into how these datasets can transform various sectors, particularly in home services and locksmith industries.
The Power of Data in Business
Data is often referred to as the new oil, and rightly so. It fuels decisions and strategies that lead to substantial organisational growth and customer satisfaction. By utilizing medical datasets for machine learning, businesses can harness the power of this data to derive actionable insights. But what does this mean in practical terms? Let’s explore.
Understanding Medical Datasets
A medical dataset is a structured collection of health-related data, which can include various information such as patient demographics, treatments, outcomes, and clinical findings. When integrated into machine learning algorithms, these datasets can illuminate crucial patterns and trends within populations, leading to more informed decision-making.
- Clinical Trials and Research: Improve the efficiency of clinical trials by predicting outcomes based on historical data.
- Predictive Analytics: Anticipate patient needs and outcomes, allowing for preemptive actions.
- Resource Allocation: Optimize the use of medical resources, improving service delivery.
Why Machine Learning Matters for Businesses
The application of machine learning in business strategies simplifies complex processes, minimizes human error, and escalates productivity. Here’s how machine learning, powered by medical datasets, can dramatically change the game:
1. Enhanced Decision-Making
With access to comprehensive datasets, businesses can make decisions backed by solid evidence rather than intuition. For instance, in the healthcare sector, understanding seasonal health trends enables hospitals to prepare better for patient influx.
2. Improved Customer Experience
Employing machine learning models can help businesses personalize services. In home services and locksmith industries, understanding customer preferences based on past data leads to tailored service offerings, drastically improving customer retention.
3. Cost Efficiency
Machine learning algorithms can identify inefficiencies within business operations. By analyzing trends within medical datasets, companies can reduce waste and optimize processes, ultimately leading to significant cost savings.
The Intersection of Home Services and Machine Learning
Home services, including locksmith solutions, stand to benefit immensely from the integration of machine learning and medical datasets. Here are compelling ways that this synergy could enhance service delivery:
Predictive Maintenance
Using machine learning, locksmiths can predict when a lock might fail based on patterns recognized through past service requests. This preemptive approach can save customers time and money while enhancing service trust.
Identifying Security Needs
By analyzing data on crime rates in various demographics, locksmiths can advise homeowners on optimal security solutions. This data-driven recommendation is invaluable in ensuring a safe living environment.
Streamlined Operations
Machine learning can automate routine tasks in home service operations, such as customer follow-ups and appointment scheduling, leading to enhanced customer satisfaction and reduced operational overhead.
Incorporating Data Ethics in Machine Learning
As businesses harness the potential of medical datasets for machine learning, it’s crucial to acknowledge data ethics. Ethical considerations must guide the use of medical data to respect privacy and maintain trust. Here are key points to consider:
1. Data Privacy
Protecting patient information is paramount. GDPR and HIPAA regulations ensure that data is used responsibly without compromising individual privacy. Businesses must implement stringent data protection measures.
2. Bias Avoidance
Machine learning models can inadvertently perpetuate biases present in datasets. It is vital to train models on diverse datasets to avoid skewed outcomes that could negatively affect underserved populations.
3. Transparency and Accountability
Businesses should be transparent about how they use data and the implications of their machine learning strategies. This fosters a culture of accountability and builds trust with consumers.
Future Trends in Machine Learning and Home Services
As we continue to explore the intersection of technology and business, certain trends are poised to shape the future landscape:
- AI-Driven Customer Interactions: Expect a rise in AI chatbots capable of delivering quick and personalized customer assistance.
- Smart Home Integration: The rise of IoT devices will lead to increased demand for locksmiths knowledgeable in smart lock solutions.
- Advanced Analytics: Businesses will rely more on predictive analytics, making informed decisions that cater to current market demands.
Conclusion: Embracing the Change
The fusion of medical datasets for machine learning with traditional home services and locksmith industries heralds a new era of efficiency and innovation. By embracing these changes, businesses not only enhance their service offerings but also ensure that they stand out in a competitive landscape.
As the business world continues to evolve, it is essential to stay ahead of the curve by adopting new technologies and methodologies. The future holds incredible potential for those willing to harness the power of data and machine learning, leading to groundbreaking advancements and unparalleled success.
In conclusion, whether you’re a small service provider or a large enterprise, leveraging the insights from medical datasets can provide a competitive edge. Your ability to use data effectively will certainly define your business trajectory in the coming years.
medical dataset for machine learning