Ultimate Guide to Labeling Training Data for Businesses
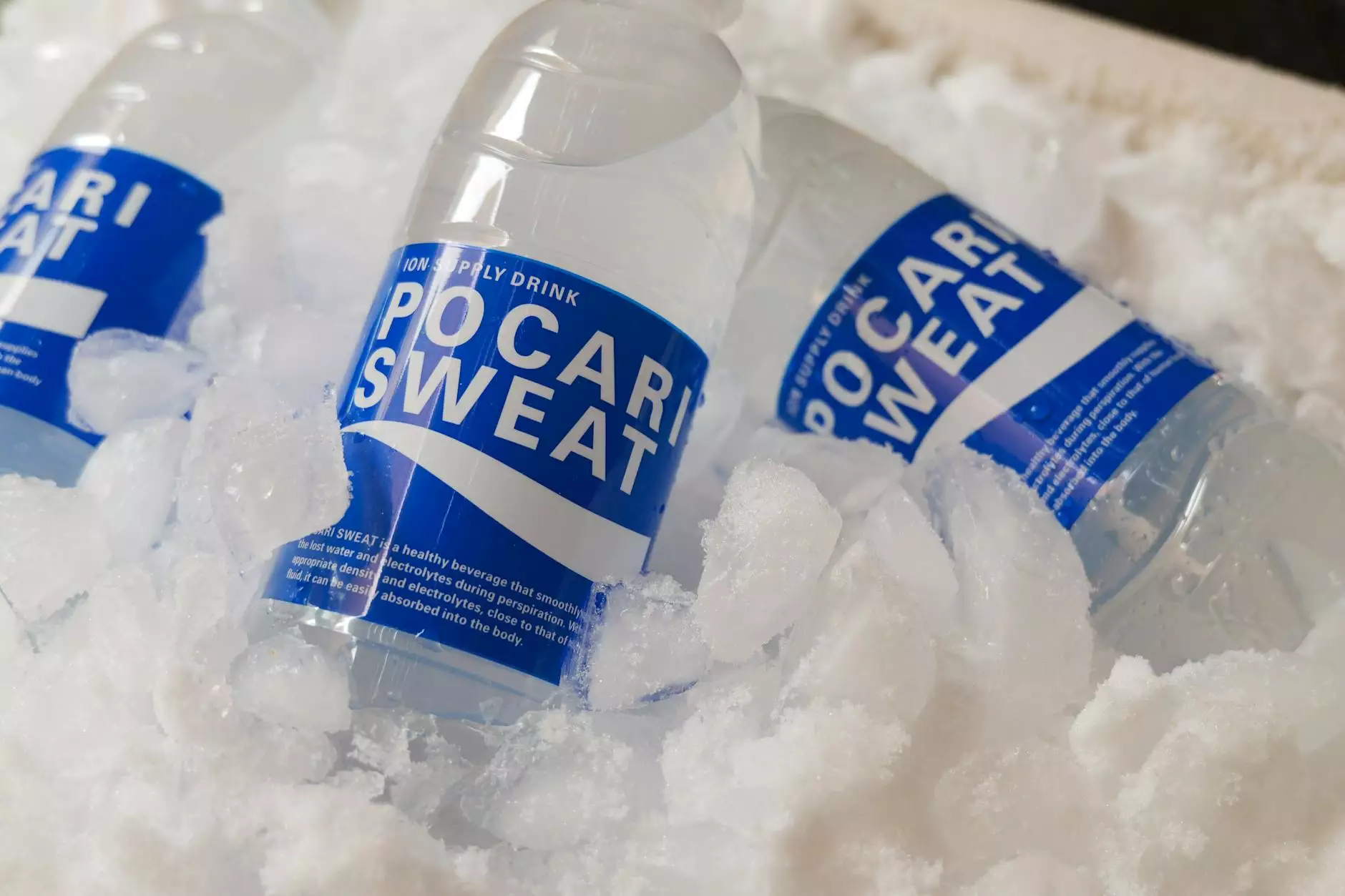
In today's data-driven world, labeling training data plays a crucial role in the success of various industries, especially those integrating artificial intelligence (AI) and machine learning (ML) technologies. This article delves deep into the significance of precise data annotation and elucidates how businesses can harness the power of data annotation tools and platforms, like those provided by Key Labs AI, to enhance their operations and outcomes.
What is Labeling Training Data?
Labeling training data refers to the process of tagging or annotating raw data to make it understandable for machine learning algorithms. This process involves categorizing and classifying data so that AI systems can learn from it effectively. Labeling can be applied to various data types, including:
- Images: Objects, faces, or elements within images can be tagged.
- Text: Sentiments, intents, and entities in written documents can be annotated.
- Audio: Distinguishing sounds, voices, or commands in audio files.
- Video: Highlighting specific activities or objects in video content.
Why Labeling Training Data is Essential for Businesses
The process of labeling training data is vital for several reasons:
1. Improved Accuracy of Models
Accurate labeling ensures that machine learning models receive the correct information needed to make predictions and decisions. High-quality labeled data is the foundation of AI accuracy. Without it, models may underperform, leading to flawed outcomes that can have significant ramifications for businesses.
2. Better Decision-Making
With correct labels, companies can glean valuable insights from their data, leading to well-informed decision-making processes. Accurate annotations in data enable businesses to understand trends, customer preferences, and operational inefficiencies.
3. Enhanced User Experiences
Labeling training data directly impacts the end-user experience. For instance, correctly labeled product recommendations can help tailor user interactions, leading to higher customer satisfaction and retention.
Key Components of Effective Labeling
To successfully implement labeling training data processes, it's essential to focus on the following components:
1. Clarity in Instructions
Clear and concise labeling instructions are paramount. Annotators must understand the goals of the labeling task and the specific categories to use. This clarity ensures consistency across the labeled data.
2. Quality Assurance Checks
Implementing a robust quality assurance framework helps in identifying and correcting errors in labeled data. Regular audits, peer reviews, and feedback loops are essential for maintaining high-quality outcomes.
3. Continuous Improvement
The data annotation landscape is ever-changing. Regularly updating labeling guidelines and practices will help improve accuracy and ensure alignment with business objectives.
Data Annotation Tools and Their Importance
The right tools can significantly enhance the efficiency of the labeling process. Key Labs AI offers various data annotation tools designed for businesses to streamline this process. Here’s why these tools matter:
1. Efficiency and Speed
Automation in data annotation tools accelerates the labeling process. This means businesses can process more data in less time, improving their ability to iterate and refine AI models rapidly.
2. Scalability
As businesses grow, their data needs increase. Tools like those from Key Labs AI are designed to scale seamlessly, allowing for the efficient handling of vast datasets without compromising on quality.
3. User-Friendly Interfaces
Many data annotation platforms feature intuitive designs, reducing the learning curve for annotators. This ease of use encourages more effective participation and enhances productivity.
Strategies for Efficient Data Annotation
For businesses looking to optimize their labeling training data processes, implementing efficient data annotation strategies is key. Here are some proven techniques:
1. Leverage Crowdsourcing
Crowdsourcing enables businesses to tap into a broader pool of annotators. This approach not only reduces costs but also increases the diversity of perspectives in the labeling process, leading to richer datasets.
2. Use Pre-trained Models
In some instances, pre-trained models can assist in the labeling process by automatically annotating sections of data. These models can serve as a starting point, which human annotators can then refine for increased accuracy.
3. Train Annotators Regularly
Regular training sessions can enhance the skills and knowledge of your annotation team. Keeping annotators updated on industry best practices and tools ensures the consistency and quality of labeled data.
Challenges in Labeling Training Data
While labeling training data is essential, it also comes with its challenges. Understanding these hurdles can help businesses prepare for and mitigate potential issues:
1. Subjectivity in Labeling
Different annotators may interpret labeling instructions differently. This subjectivity can lead to inconsistencies. To combat this, clear guidelines and regular training are essential.
2. Handling Large Volumes of Data
As data grows exponentially, managing and labeling this data can become a daunting task. Leveraging automation and scalable tools can help manage this challenge effectively.
3. Time Constraints
Time is often of the essence in competitive industries. Businesses must balance the need for quality labeling with the urgency of project timelines. Streamlining processes and leveraging efficient tools can help strike this balance.
Best Practices for Labeling Training Data
To maximize the effectiveness of your labeling efforts, consider the following best practices:
1. Define Clear Objectives
Every labeling project should have clear objectives. Understanding the end goals will guide the annotation process effectively.
2. Utilize Diverse Datasets
Diverse datasets provide AI models with a breadth of information leading to more robust learning outcomes. Ensure your training data encompasses various scenarios and cases to improve generalization.
3. Implement Feedback Mechanisms
Constructive feedback loops are critical. Encourage feedback on the annotation process and outcomes to continually improve labeling practices.
The Future of Labeling Training Data
As technology advances, the way businesses approach labeling training data will evolve. Here are some anticipated trends:
1. Increased Use of AI in Annotation
The future will likely see a greater reliance on AI-powered tools to assist in the labeling process, reducing the burden on human annotators while improving speed.
2. Enhanced Collaboration Tools
Collaboration platforms will grow in importance, allowing teams to work together seamlessly, regardless of location.
3. Focus on Ethical Considerations
As data privacy concerns rise, ethical considerations in data labeling will become paramount. Transparency in data use and labeling processes will become a competitive advantage.
Conclusion
Labeling training data is not just a technical requirement; it is a strategic advantage for businesses looking to thrive in the AI landscape. By adopting systematic approaches to data annotation, leveraging advanced tools like those offered by Key Labs AI, and fostering a culture of quality and continuous improvement, businesses can unlock the full potential of their data.
Investing in high-quality labeling training data is an investment in the future success of your business. As AI technologies continue to evolve, the accuracy and relevance of your datasets will determine how effectively your models perform and how well your business adapts to changes in the market.