Unlocking the Power of Annotated Images: The Key to Data Annotation Tools and Platforms
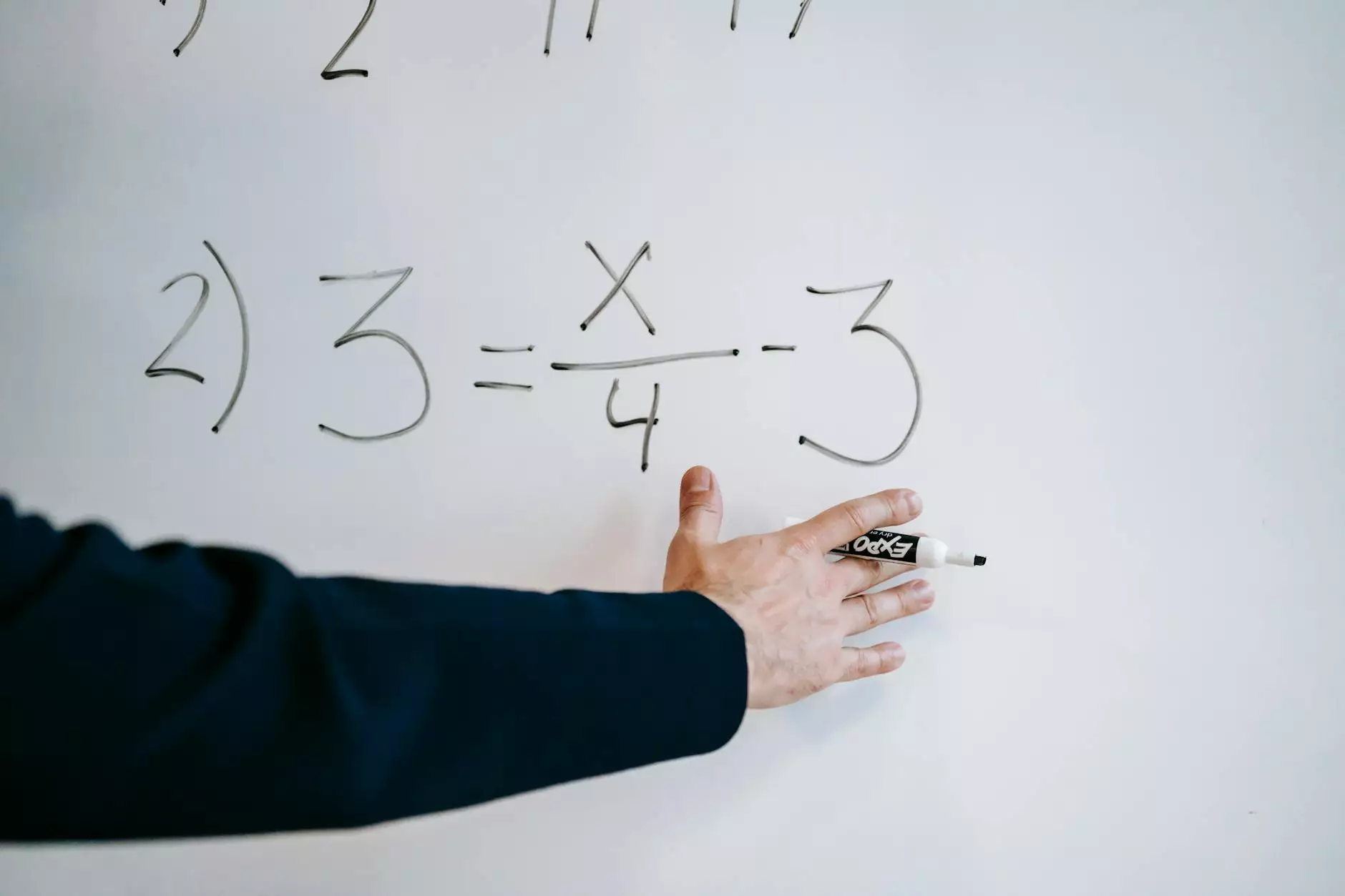
Introduction to Data Annotation and Its Significance
In today's rapidly evolving technological landscape, data annotation stands as a critical element enabling the development of intelligent systems. This article dives deep into the realm of annotated images, exploring their vital role within data annotation tools and data annotation platforms.
Data annotation refers to the process of labeling data to make it understandable for machine learning models. It is a foundational step in training AI to recognize patterns and extract insights from large datasets. Among various data types, annotated images play a paramount role, particularly in fields such as computer vision, where algorithms must interpret and understand visual information.
What Are Annotated Images?
Annotated images are photographs or graphics that have been marked with labels or tags. These annotations guide machine learning models to accurately identify and classify objects within the images. The practice of using annotated images is prevalent across several industries, including healthcare, automotive, and e-commerce.
For instance, when training a self-driving car, engineers utilize annotated images to teach the system about various objects like pedestrian crossings, traffic signals, and road signs. By providing detailed labels and boundaries within these images, we enhance the accuracy of the AI model’s understanding and decision-making processes.
The Importance of Annotated Images in Machine Learning
The integration of annotated images into machine learning pipelines significantly boosts model performance. Here are several key reasons why:
- Enhanced Model Accuracy: Models trained on well-annotated datasets demonstrate higher accuracy in predictions, leading to better overall performance.
- Reduced Bias: Comprehensive labeling helps in reducing bias, ensuring that models perform well across different demographics and conditions.
- Improved Generalization: Well-labeled examples enable the model to learn robust features, improving its ability to generalize to unseen data.
- Efficient Training Processes: With precise annotations, the training data becomes more effective, allowing quicker and more productive training cycles.
Types of Data Annotation Used with Images
Understanding the different types of data annotation used with images can help stakeholders select the right approach for their projects. Here are some common methods:
- Bounding Box Annotation: This method involves framing an object within a rectangular box, commonly used for detecting and identifying objects like vehicles in traffic imagery.
- Semantic Segmentation: This technique requires pixel-wise labeling, allowing precise delineation of objects within an image, vital for applications in medical imaging.
- Polygon Annotation: More complex objects that can't be contained in a simple rectangle may use polygon annotations, allowing for precise outlining of irregular shapes.
- Landmark Annotation: Used primarily for facial recognition, this approach involves marking specific points of interest such as the eyes, nose, and mouth.
- 3D Point Cloud Annotation: This is essential for applications involving three-dimensional data, often used in robotics and autonomous vehicles.
Choosing the Right Data Annotation Tool
When it comes to implementing a data annotation platform, choosing the right tool is crucial. Here are several factors to consider:
- User-Friendly Interface: A tool with an intuitive design minimizes the learning curve for users and enhances efficiency.
- Scalability: The platform should easily scale according to project requirements, accommodating growing dataset sizes.
- Collaboration Features: Effective tools allow multiple users to collaborate simultaneously, streamlining the annotation process.
- Integration Capabilities: The ability to integrate with existing data systems and workflows is crucial for seamless operations.
- Quality Assurance Mechanisms: Tools that offer built-in quality checks can ensure the precision and reliability of annotated images.
The Future of Annotated Images in AI
The future of annotated images in artificial intelligence and machine learning is undoubtedly promising. As technology advances, we can expect innovations that will:
- Automate Annotation Processes: AI itself will be used to automate parts of the annotation process, lowering costs and speeding up the workflows.
- Improve Accuracy with Active Learning: Utilizing active learning methodologies will enable models to suggest the most informative data points for annotation, thus continually improving the dataset.
- Enable Real-Time Data Annotation: Future tools will allow for real-time annotation of images, significantly benefiting industries that rely on immediate feedback.
- Support a Broader Range of Formats: We can anticipate support for new formats beyond traditional image files, including video annotations, which will further expand the potential applications.
Best Practices for Annotating Images
To maximize the effectiveness of annotated images, it's essential to adopt best practices throughout the data annotation process:
- Define Clear Guidelines: Establish clear guidelines for annotators to follow, ensuring consistency and clarity in the annotation process.
- Train Your Annotators: Providing proper training for annotators helps maintain high quality and accuracy in the labeled data.
- Conduct Regular Reviews: Implementing regular quality checks ensures that the annotations meet the required standards.
- Use Diverse Data Sources: Incorporate diverse datasets to support the model’s ability to generalize across different cases and conditions.
- Prioritize User Feedback: Involve users in providing feedback on the annotation process to identify areas of improvement and optimize workflows.
Conclusion: Harnessing the Power of Annotated Images
In conclusion, the world of annotated images is pivotal for advancing artificial intelligence, particularly concerning data annotation tools and data annotation platforms. As the demand for high-quality training data intensifies, investing in robust annotation strategies and tools becomes essential.
Businesses looking to excel in AI-driven initiatives must leverage the power of annotated images to create accurate, reliable, and effective models. By adhering to best practices, utilizing appropriate tools, and embracing emerging trends, organizations can significantly enhance their operational performance and drive innovation.
As we explore the future of data annotation, one thing remains clear: the significance of annotated images will only grow, paving the way for breakthroughs in machine learning and artificial intelligence.